What is Cognitive Document Processing?
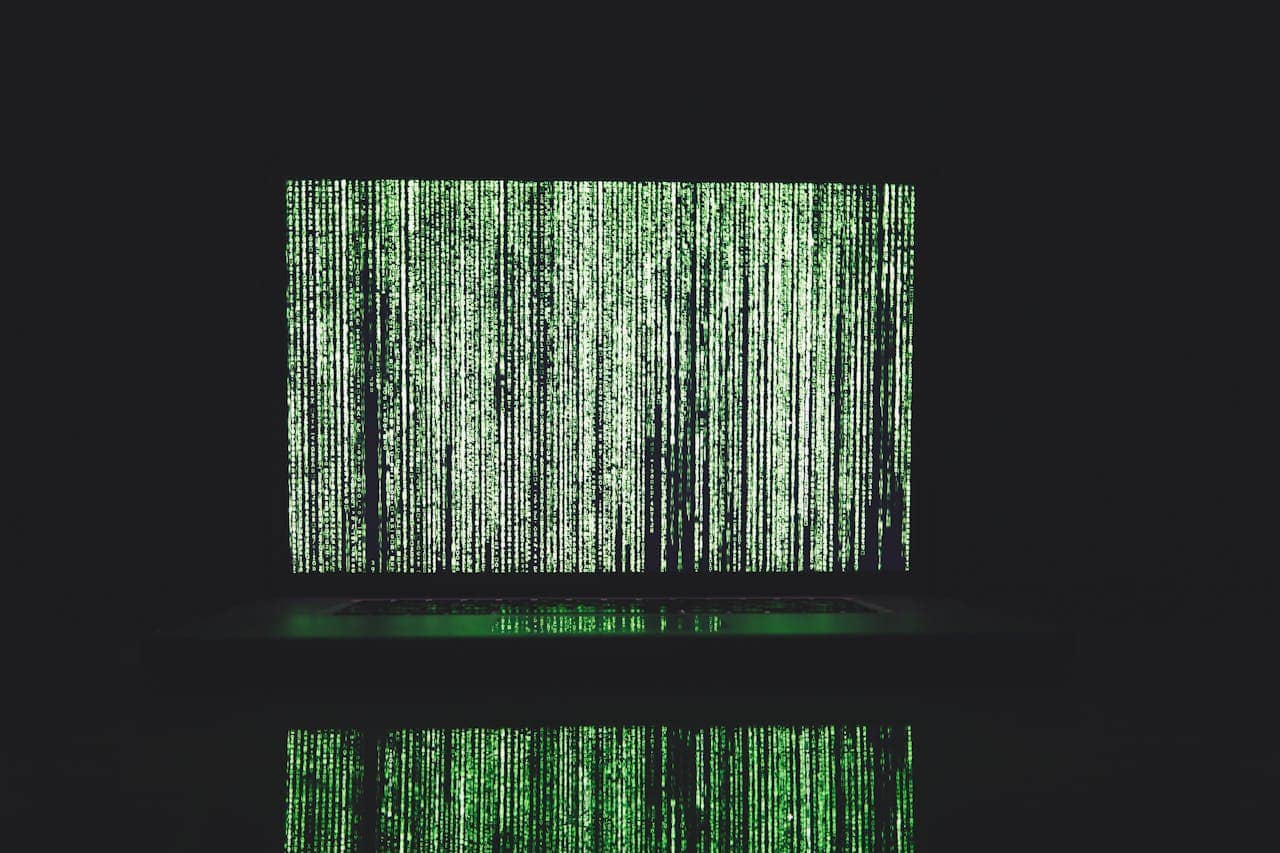
Cognitive document processing is a transformative approach that applies artificial intelligence (AI) to interpret and manage unstructured data within documents, much like the human brain processes information. It uses a combination of techniques, such as natural language processing (NLP), machine learning (ML), and sometimes robotic process automation (RPA), to automate the extraction, classification, and analysis of data from various types of documents. This technology expedites decision-making processes and significantly reduces the manual effort typically involved in handling documents.
By transforming unstructured data into structured, actionable insights, cognitive document processing allows organizations to streamline their document workflows. It supports a range of applications, from simple data entry to complex information extraction and decision-making tasks. It's a step beyond traditional document processing, offering a level of understanding and accuracy that mimics human cognition but operates at a greater speed and consistency.
The core advantage of cognitive document processing lies in its ability to adapt and learn from the data it processes, continuously improving its accuracy and efficiency over time. By harnessing this powerful AI-driven approach, businesses can manage their documents more effectively, leading to operational efficiencies, cost savings, and enhanced compliance with regulations, ultimately giving them a competitive edge in the digital age.
Understanding Cognitive Document Processing
Cognitive Document Processing (CDP) significantly enhances the way businesses manage and interpret vast quantities of documents. By integrating artificial intelligence, it allows for a deeper level of learning and comprehension, modifying traditional methods through the application of cognitive functions.
Fundamentals of Cognitive Document Processing
Cognitive Document Processing utilizes AI to extract, interpret, and manage data from a variety of document types. This method involves several key stages:
- Data Acquisition: This first stage involves collecting documents from numerous sources.
- Data Extraction: AI algorithms then extract relevant data from these documents.
- Comprehension: The system applies natural language processing (NLP) to understand the context and meaning within the data.
- Data Integration: Finally, the extracted and comprehended data is transformed and integrated into the pertinent systems for decision-making purposes.
CDP systems leverage machine learning models, continually improving their accuracy and efficiency as more data is processed.
Cognition and AI in Document Processing
The role of cognition in document processing lies in mimicking human-like understanding:
- Artificial Intelligence (AI): It forms the core of cognitive document processing, enabling systems to make sense of unstructured data.
- Learning: Machine learning algorithms within CDP adapt and evolve, improving their capabilities through experience.
- Natural Language Processing (NLP): A subfield of AI, NLP allows the system to interpret and analyze human language, turning text into structured data.
Together, these cognitive technologies enable businesses to automate complex document-processing tasks that traditionally require human intervention, accelerating decision-making and streamlining workflows.
Technologies Behind Cognitive Document Processing
Cognitive Document Processing (CDP) integrates various advanced technologies that enable the automation of document interpretation. These technologies help machines to understand documents in a human-like manner, transforming unstructured data into actionable insights.
Machine Learning and Natural Language Processing
Machine Learning (ML) and Natural Language Processing (NLP) are the foundational technologies that determine the efficacy of CDP systems. Machine learning algorithms are trained on vast datasets to recognize patterns, interpret data, and make decisions with minimal human intervention. When combined with NLP, these systems acquire the capability to understand and process human language within documents. Azure AI, for instance, employs these technologies in services like the Form Recognizer to extract key-value pairs and tables from forms.
Optical Character Recognition (OCR) and Computer Vision
Optical Character Recognition (OCR) technology is integral to CDP, allowing the conversion of different types of documents, including scanned paper documents and images, into editable and searchable data. Computer Vision extends this capability; it aids in understanding the content and context of images and scanned documents, such as layout and spatial relationships. OCR and Computer Vision work in tandem to digitize and interpret the visual information from documents, which is necessary for further processing by AI-driven systems.
Operational Benefits and Use Cases
Cognitive document processing technology offers significant operational advantages by enhancing efficiency and accuracy in managing both structured and unstructured data. These improvements are evident in specific applications across various industries, where the handling of invoices, forms, and contracts is streamlined, leading to reduced operational costs and heightened productivity.
Boosting Efficiency and Accuracy
- Efficiency: Cognitive document processing automates repetitive tasks, allowing for quicker completion and freeing up personnel for more complex roles. For example, in the accounts payable process, automation can reduce the time taken to process invoices, resulting in faster payment cycles.
- Accuracy: By leveraging artificial intelligence, such systems increase the accuracy of data extraction from documents, minimizing the likelihood of errors that can occur with manual entry. In contexts where precision is paramount, like contract analysis, this contributes to reducing risks and improving compliance.
Applications in Various Industries
- Healthcare: Healthcare providers utilize cognitive document processing to manage patient forms and records, ensuring that crucial information is accurately captured and available for treatment decisions.
- Insurance: The insurance sector employs these systems to process claims and evaluate policy documents rapidly, aiding in swift claim resolutions and customer service.
- Financial Services: For financial institutions, automating the extraction and analysis of data from various financial documents, like accounts statements and loan applications, enhances decision-making processes.
In these use cases, cognitive document processing not only streamlines workflows but also supports organizations in navigating the complexities of data management, ensuring they can make informed decisions while optimizing their resource allocation.
Challenges and Future Trends
As organizations increasingly adopt cognitive document processing, certain obstacles persist while new trends hint at a significant transformation in how documents will be managed in the future. This section will examine the prevalent challenges and the anticipated evolution within the field.
Overcoming Common Obstacles
Integration with existing systems poses a notable challenge for cognitive document processing solutions. Organizations face difficulties in melding new cognitive technologies with legacy systems, often resulting in scalability issues. Errorsin document handling may arise from mismatches between the artificial intelligence models and the varying structure of documents. Ensuring robust verification processes is critical to mitigate these errors and enhance the accuracy of data extraction.
In the context of digital transformation, companies must also navigate the complexities that come with transitioning from manual to automated processes. It is essential for cognitive automation solutions to be adaptive and cater to an ever-changing digital environment.
The Evolution of Cognitive Document Processing Systems
Cognitive document processing systems are anticipated to see remarkable advancement driven by deep learningalgorithms. These systems are evolving to handle a wider array of document types with greater precision.
Key Trend | Potential Impact |
---|---|
Deep Learning Enhancement | More accurate and nuanced understanding of complex documents. |
Scalable Solutions | Greater flexibility in processing document volumes of varied sizes. |
Advanced Error Handling | Improved ability to self-correct and learn from processing anomalies. |
The future of document processing solutions leans towards comprehensive cognitive automation solutions, which will not only understand and categorize data but also predict and recommend subsequent processes. This is set to revolutionize efficiency and decision-making within organizations, propelling them toward a more agile and data-driven future.