Extracting Data from Financial Statements: Key Techniques
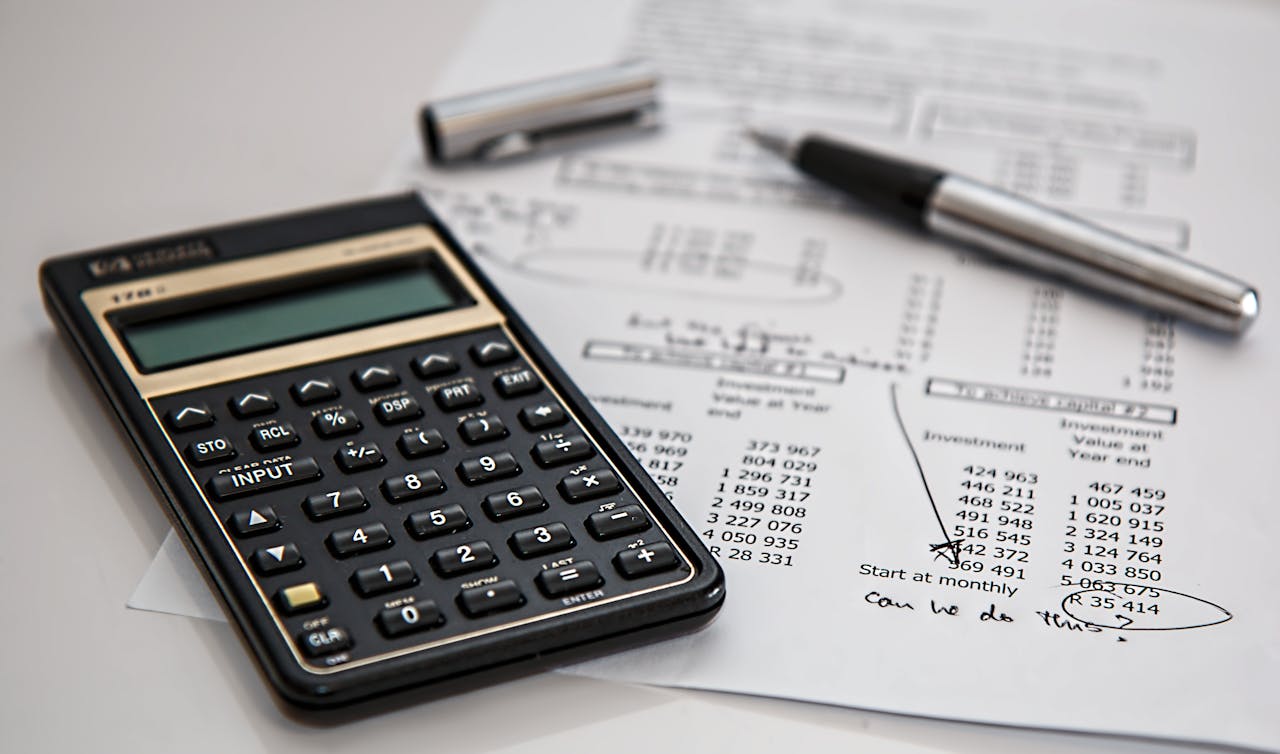
Extracting data from financial statements is a crucial process in financial analysis, reporting, and strategic planning. The meticulous transformation of data from its original form, often unstructured or semi-structured, into a structured format enhances the ease of analyzing financial health and business performance. Precise and structured data serves as the bedrock for all levels of financial decision-making, underpinning the accuracy and reliability of insights gleaned from financial statements.
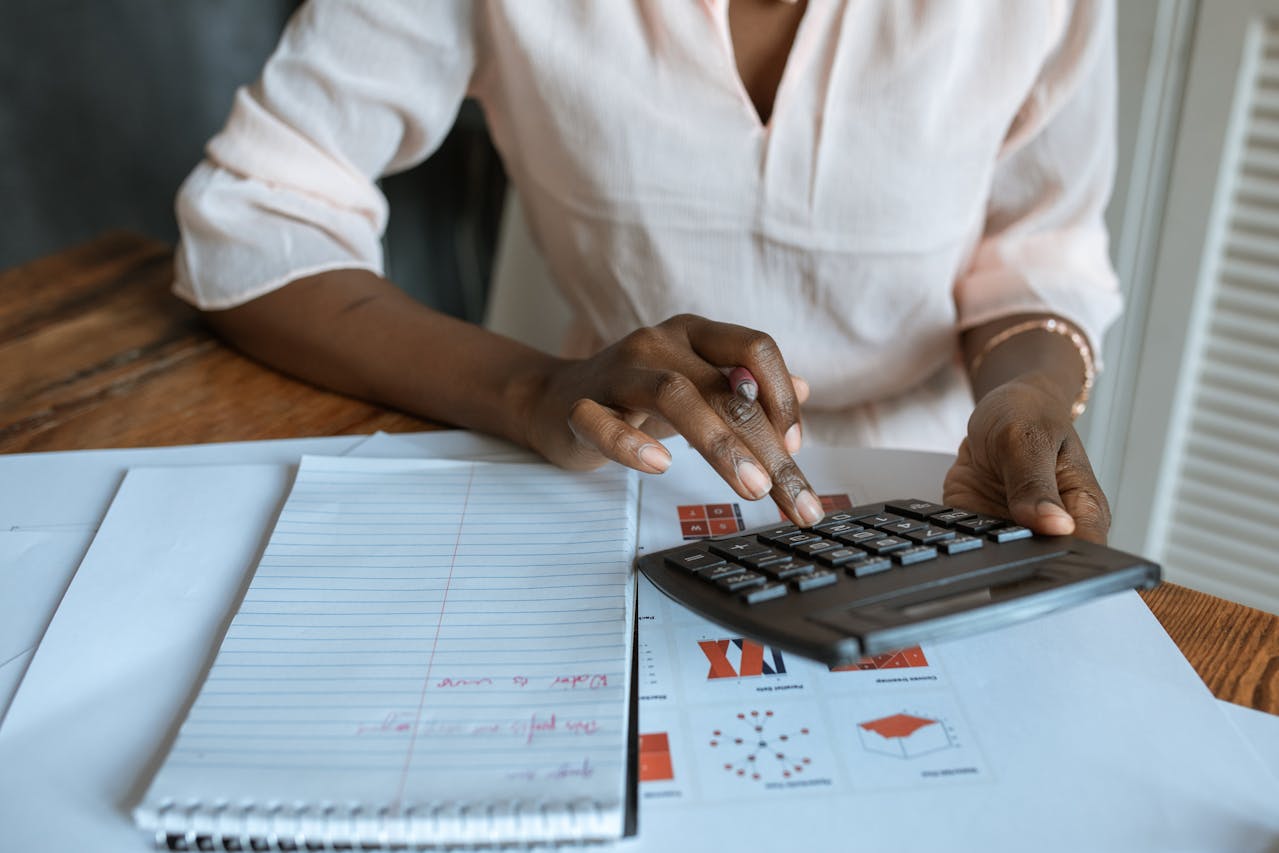
Data extraction procedures vary, but common to all is the application of advanced algorithms and artificial intelligence technologies. These technologies process raw data from financial documents, such as income statements, balance sheets, and cash flow statements, to distill essential financial metrics and information. The evolution from traditional manual methods to automated, AI-powered workflows has greatly reduced human error, elevated data quality, and saved significant time and resources.
Financial data extraction enables stakeholders to acquire timely and accurate information, simplifying subsequent analysis and the derivation of actionable business insights. A robust data extraction strategy guarantees that only relevant and crucial information is captured, perfectly positioning businesses to understand their financial narratives and make informed decisions with confidence.
Understanding Financial Statements
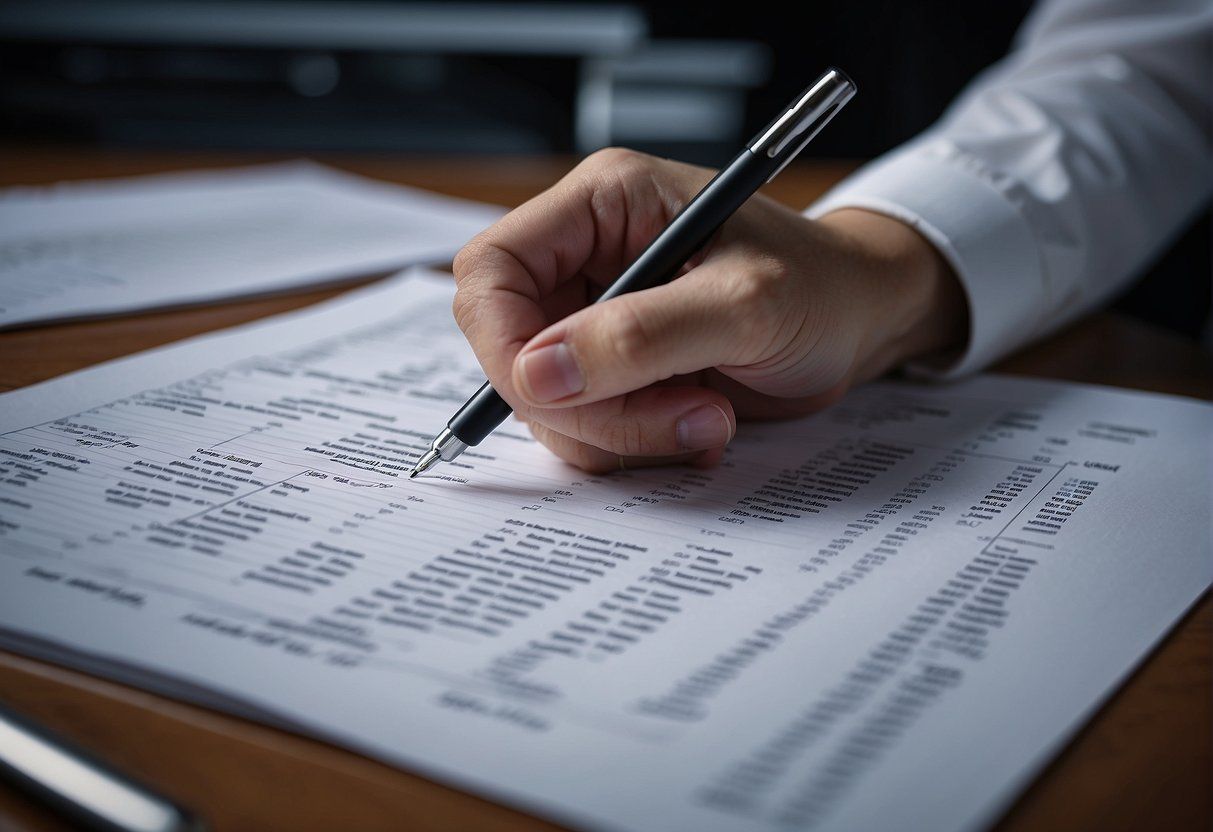
Before diving into the specifics, it's essential to comprehend that financial statements provide a formal record of the financial activities of an entity, reflecting its financial health and performance to investors.
Components of Financial Statements
Financial statements predominantly consist of three key components:
Balance Sheet: It provides a snapshot of an entity's financial condition at a specific point in time. It comprises:
- Assets: Resources owned by the company, like cash, inventory, and property.
- Liabilities: Obligations owed to creditors, including loans and accounts payable.
- Equity: The residual interest in the assets of the entity after deducting liabilities, often referred to as shareholder's equity.
Income Statement: This statement, also known as the profit and loss statement, outlines the company's financial performance over a period, detailing:
- Revenue: Income from sales of goods or provision of services.
- Expenses: Costs incurred in the process of earning revenue, such as cost of goods sold (COGS) and operational expenses.
- The bottom line of this statement is the Net Income, which is the profit or loss after all revenues and expenses have been accounted for.
- Cash Flow Statement: It tracks the flow of cash in and out of the business in three activities: operational, investing, and financing. It provides insights into the liquidity and viability of a company.
Importance of Financial Data Accuracy
Accurate financial data is the bedrock for assessing an entity's financial health and making sound investment decisions. Investors rely on verified figures to gauge a company’s performance, potential for growth, and the management's ability to efficiently run operations. Discrepancies or errors in financial statements can lead to incorrect assessments of a company's value and could affect decision-making processes pertaining to investments, lending, or management strategies. Accurate financial statements are also essential for regulatory compliance and maintaining the trust of stakeholders.
Technologies for Data Extraction
The landscape of financial data extraction has transformed with the advent of advanced technologies. They not only offer greater accuracy but also automate processes that were previously manual and time-consuming.
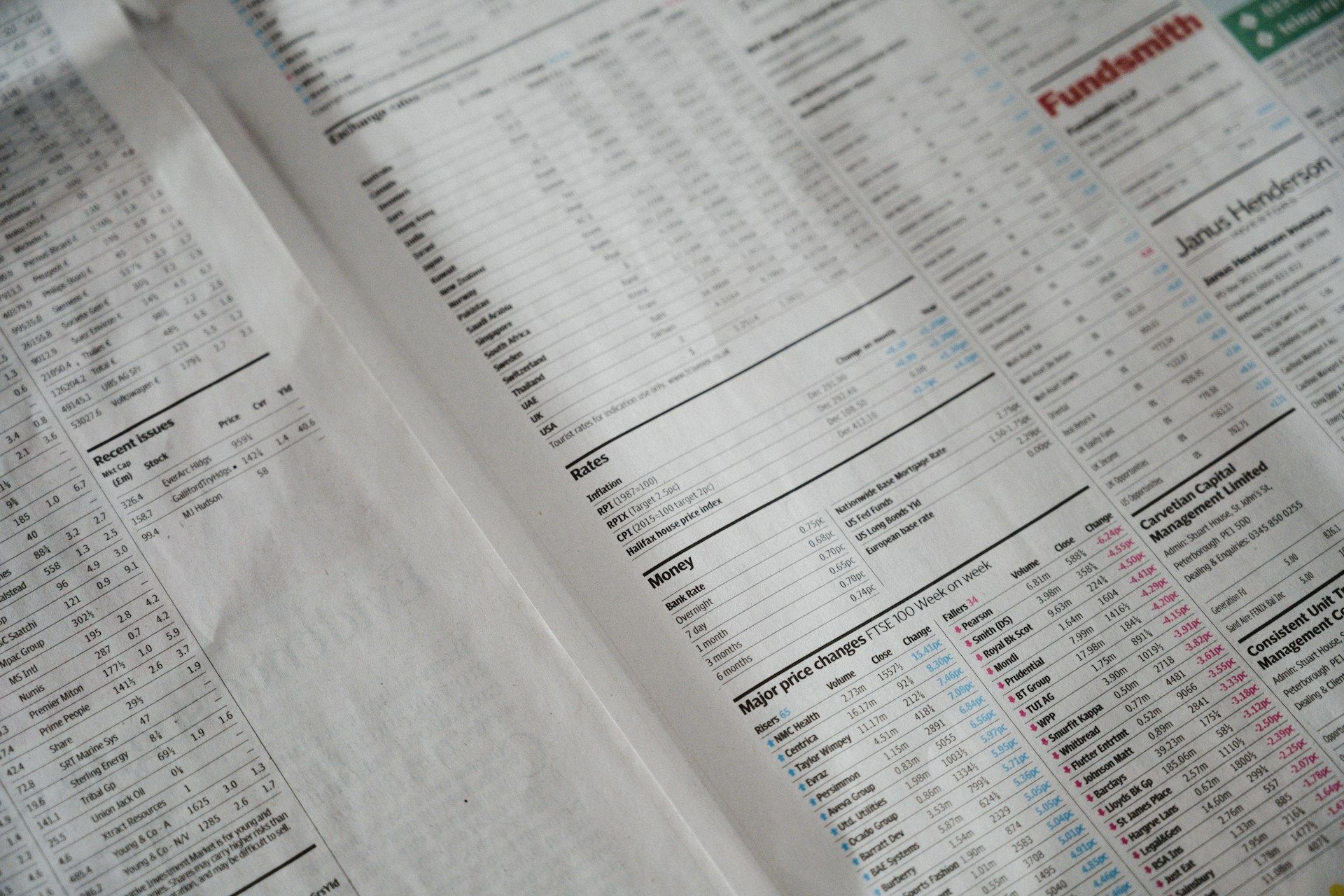
Optical Character Recognition (OCR) in Finance
OCR technology plays a pivotal role in financial document analysis by converting different types of documents, such as scanned papers or PDFs, into editable and searchable data. This is highly beneficial in finance for extracting data from bank statements, invoices, and receipts. Accuracy and speed are the primary advantages, as OCR reduces human error and expedites data retrieval.
Intelligent Document Processing (IDP)
IDP technology elevates OCR capabilities by incorporating machine learning (ML), natural language processing (NLP), and computer vision. It not just reads the text, but also understands the content, context, and semantics within financial documents. Through its AI-driven approach, IDP ensures that data extraction is not only accurate but also contextually relevant, facilitating better decision-making.
Machine Learning and AI Enhancements
The integration of ML and AI further enhances data extraction software, enabling automation of complex tasks. By learning from data patterns and decisions, the technology continually improves, leading to more precise financial data extraction. This automation relieves financial professionals from repetitive tasks, allowing them to focus on analysis and strategy. AI systems are designed to understand financial terminology and concepts, increasing the effectiveness of data extraction and analysis.
The incorporation of these technologies marks a significant shift in the efficiency and reliability of financial data analysis, streamlining the extraction process while ensuring the integrity of the data collected.
Integration and Automation in Data Extraction
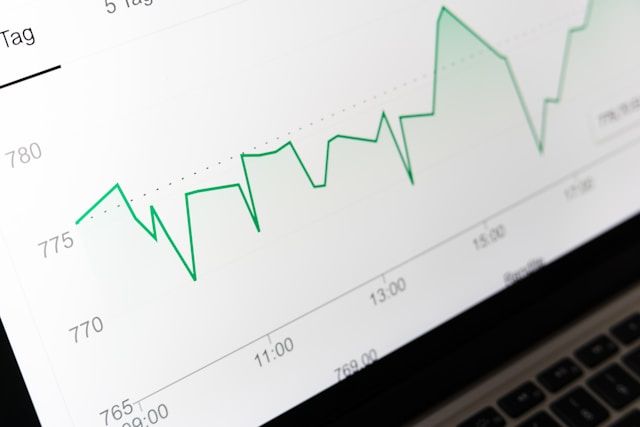
Integrating advanced software solutions and automating the workflows are critical in enhancing the efficiency of data extraction from financial statements. They enable a higher degree of accuracy in transforming raw financial data into structured insights invaluable for decision-making.
Seamless Integration with Accounting Systems
Financial data extraction tools must seamlessly integrate with existing accounting systems and Enterprise Resource Planning (ERP) software to ensure data continuity and quality. These systems often come with an Application Programming Interface (API) which allows for effortless connection between different software applications. For instance, a data extraction platform might connect with an ERP system, enabling it to directly access and extract necessary data points such as revenue, costs, and balance sheet items.
Automating Data Extraction Workflows
Automation in the context of data extraction implies the use of Artificial Intelligence (AI) to replicate manual data entry and analysis tasks. Automating data extraction workflows offers significant benefits:
- Efficiency: Reduces time needed to collect and process data from financial documents.
- Data Quality: Minimizes human error, enhancing the accuracy of financial analysis.
- Scalability: Automation tools can handle increased volumes of data without a proportional increase in time or resources.
- Insights for Decision-Making: Streamlined extraction of data feeds into analytical models, providing real-time insights for strategic decisions.
Automated data extraction systems use AI to recognize and process diverse formats, whether they are digital PDFs or scanned paper documents. These systems learn and adapt, improving their ability to identify and extract relevant information over time, thus continually improving the workflow.
Challenges and Best Practices in Financial Data Extraction
Extracting data from financial statements presents a series of challenges that need strategic approaches to overcome. Best practices in this field are essential for achieving accurate financial analysis and reporting.
Handling Voluminous and Complex Data
Financial statements often contain a vast volume of complex data. Efficiently processing this volume can be inefficientand slow if not managed properly. Businesses should implement robust data management systems that can handle large datasets with ease. Using automation tools reduces the risk of human error that is prone to errors when dealing with such complexities.
Ensuring Data Integrity and Compliance
The integrity of financial data is paramount. Any errors in data can lead to misguided decisions and potential compliance issues. Companies must clean and validate data to ensure that it is accurate and usable. To meet regulatory compliancestandards, it’s crucial to have a system that can track data lineage and audit trails. This ensures that all financial data extraction methods adhere to industry regulations.
Addressing the Limitations of Manual Methods
Manual data extraction is a labor-intensive process that is costly and prone to human error. Best practices involve transitioning toward automation and machine learning technologies that can process complex datasets more accurately and swiftly. Automation not only minimizes the risk of error but also increases efficiency by freeing up human resources for tasks that require more critical analysis and decision-making.