What is Automated OCR Data Capture?
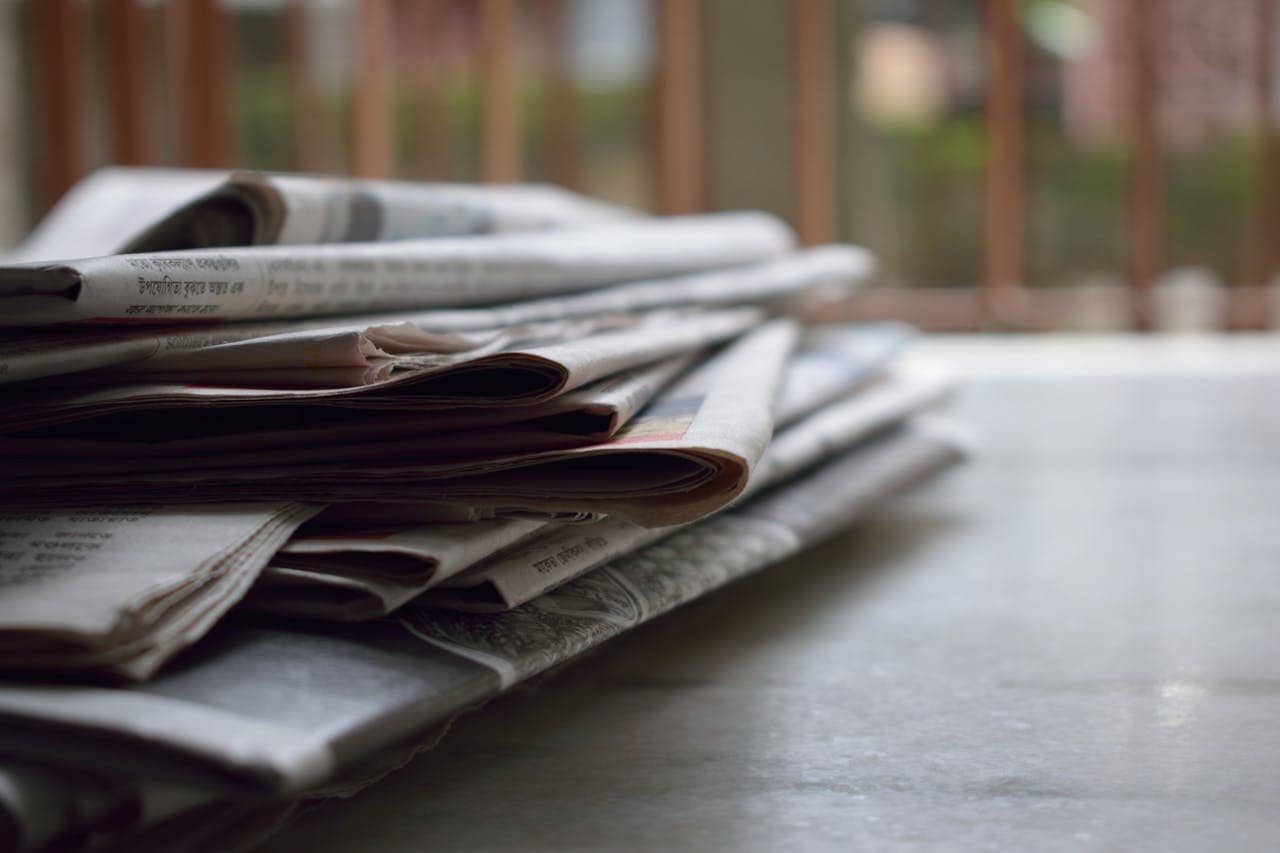
Automated OCR Data Capture is a transformative technology that allows computers to interpret and convert different types of documents, including scanned papers, PDF files, or images into editable and searchable data. This process leverages Optical Character Recognition (OCR), a subset of AI technology, to enable the efficient digitization of text. Businesses and organizations employ this technology to streamline data entry, reduce manual errors, and save time by automating the extraction of information from various documents such as invoices, forms, and receipts.
By automating the process of data capture, organizations can rapidly process large volumes of information with increased accuracy. OCR technology scans the text in documents and converts it into a format that is easily editable and searchable by a computer. This capability is particularly beneficial in environments heavy with paperwork, where it can dramatically improve the efficiency of data management processes.
The integration of OCR within data capture solutions is a testament to the advancements in AI, as it increasingly becomes more sophisticated in recognizing various fonts and handwriting styles. The extracted data from OCR can be directly fed into databases or other systems, enabling seamless data flow and supporting data-driven decision-making. This technology forms a critical part of modern information management strategies, driving productivity by allowing more focus on analysis and interpretation rather than on data entry.
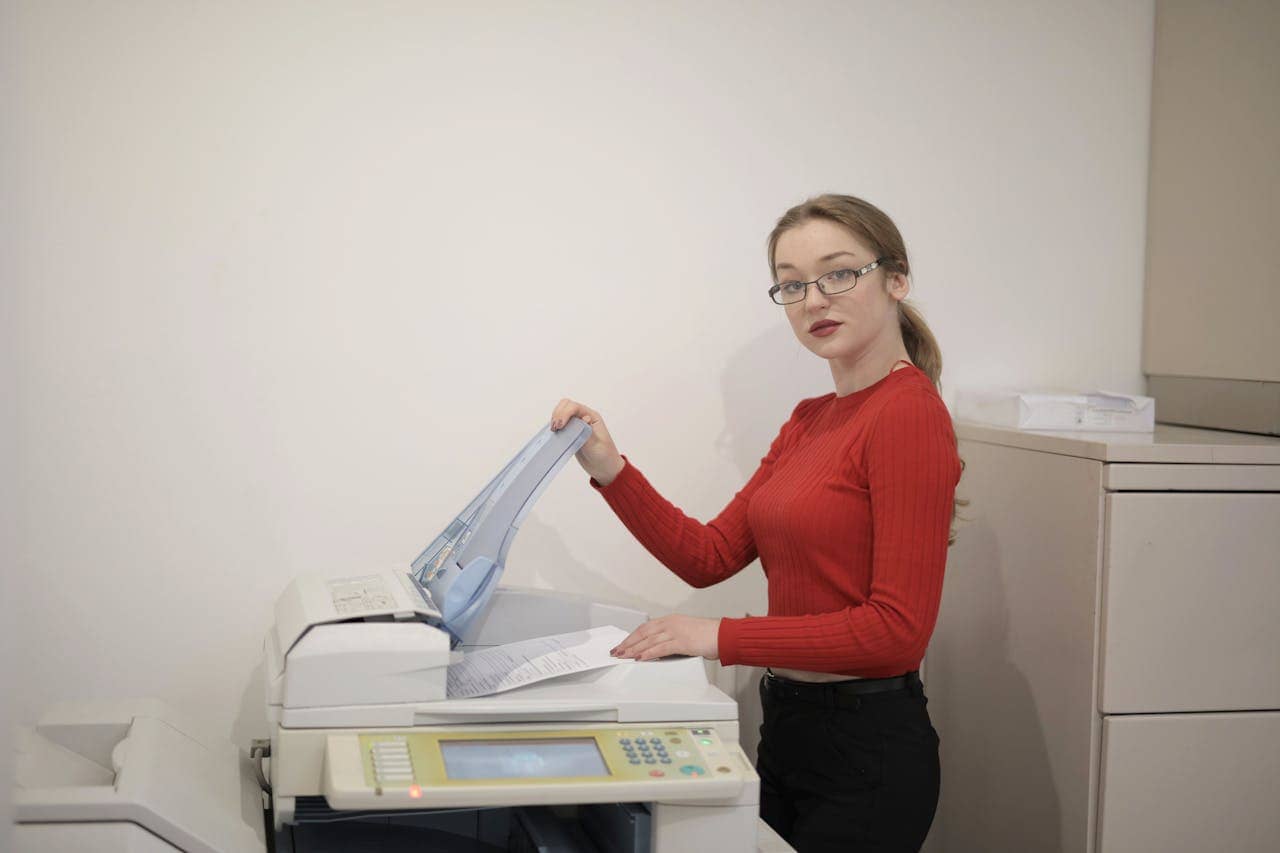
Understanding OCR and Its Advancements
Optical Character Recognition, or OCR, has evolved significantly from its origins, becoming a vital component in modern data extraction. This section explores its history and current standing, particularly how it has assimilated machine learningtechniques to enhance functionality.
History and Evolution of OCR
OCR technology's roots can be traced back to the early 20th century, primarily used as a tool to aid the visually impaired. The evolution of OCR has seen it transform from basic character recognition to sophisticated software capable of interpreting an array of fonts and styles with high accuracy. The timeline of OCR reflects a shift from mechanical devices to digital solutions that seamlessly integrate with various computer systems, enabling the automatic conversion of different types of documents into machine-readable text.
OCR and Machine Learning
The integration of machine learning into OCR systems has been a game changer. Machine learning, a subset of AI, allows OCR tools to improve with experience, increasing both accuracy and the range of characters and languages recognized. Utilizing algorithms that learn from data, these advanced OCR systems can now accurately process complex documents such as invoices, receipts, and handwritten notes, converting them into structured data formats. This synergy has expanded OCR's applications, contributing significantly to fields like data management and automation.
The Process of Automated OCR Data Capture
Automated Optical Character Recognition (OCR) Data Capture streamlines the conversion of various document types into editable and analyzable digital data, improving accuracy and efficiency in data entry and management.
Capturing Data from Various Sources
The initial step in the automated OCR data capture process involves gathering data from diverse sources. These sources can include paper documents, invoices, receipts, and forms, amongst others. This stage is vital as it lays the groundwork for accurate data extraction.
- Documents: Paper-based or digital images.
- Automate: Use of software to scan and import documents.
Extraction and Interpretation
Upon capturing images of the documents, OCR technology extracts text from these images. This phase manages both structured data (like forms or tables) and unstructured data (like free-form written text).
- Extraction: Recognition and conversion of text-based images into digital text.
- Accuracy: Advanced algorithms ensure precision in interpreting data.
- Automation: Reduces manual effort and expedites the process.
Verification and Validation
Following extraction, the obtained data undergoes a verification and validation process. This is crucial for ensuring the accuracy and reliability of the captured data before it moves into the organization's database or a third-party system.
- Accuracy: Critical to validate OCR results against original documents.
- Capture: Confirmation that all relevant data fields have been accurately captured.
- Automation: Potentially includes automated checks for common mistakes or inconsistencies.
The automation of OCR data capture marks a significant advancement from traditional manual data entry, providing organizations with a means to handle documents more quickly and with greater precision.
Applications and Use Cases in Industry
Automated OCR data capture has facilitated transformation across various industries by optimizing the processing of documents and data entry. Its integration with systems like Enterprise Resource Planning (ERP) significantly enhances data management and accuracy.
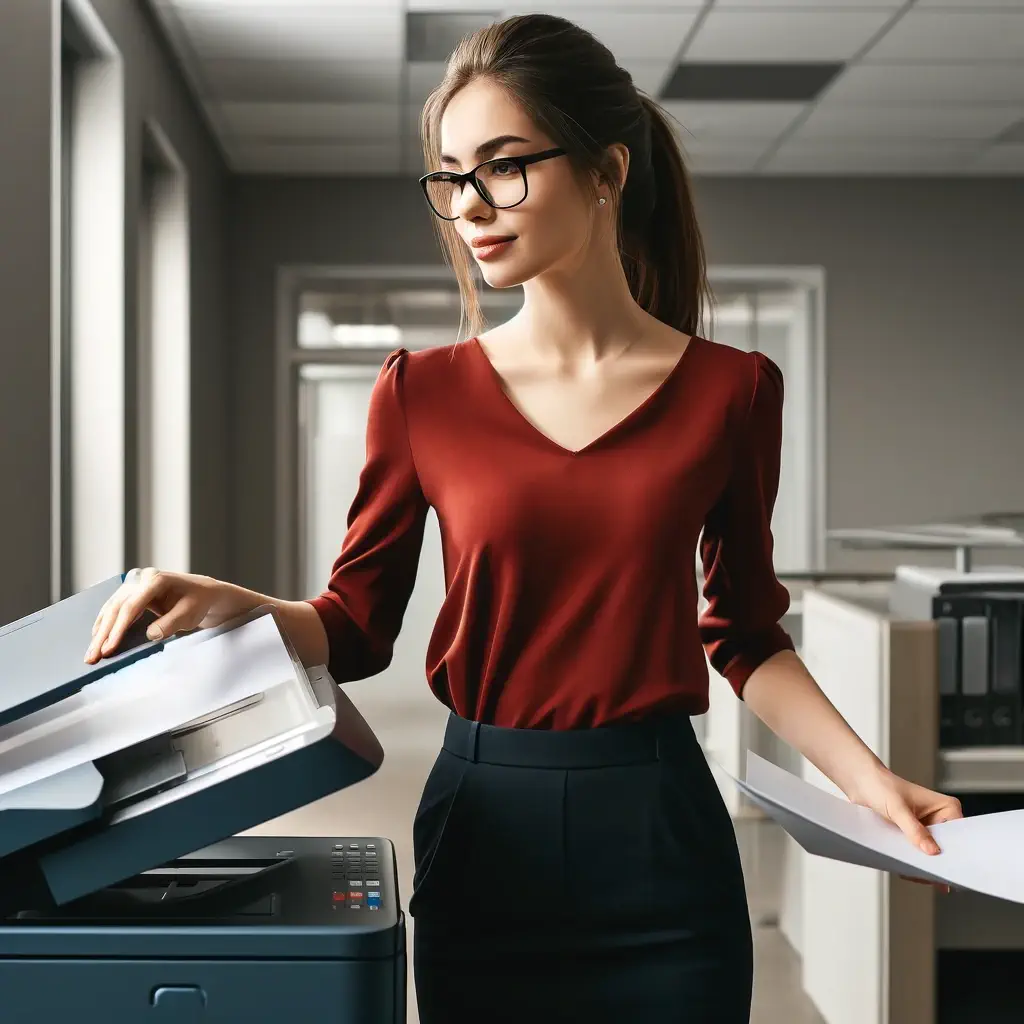
Automated Invoice Processing
In accounting and finance, Automated Invoice Processing has become a cornerstone of efficient Accounts Payabledepartments. By leveraging OCR Data Capture and Intelligent Document Processing (IDP), companies can extract data from invoices, reducing manual entry errors and accelerating payment cycles. This technology seamlessly integrates with ERP systems, leading to more timely and accurate financial reporting.
Healthcare and Insurance Document Handling
The healthcare sector utilizes OCR to manage patient records and insurance claims with higher precision and confidentiality. Healthcare providers and insurance companies benefit from the amalgamation of OCR data capture with IDP solutions, streamlining the management of sensitive documents such as patient ID forms and insurance paperwork, thus ensuring compliance and improving patient care.
Logistics and Order Management
OCR technology is integral in logistics for automating the extraction of data from shipping documents and receipts. It facilitates real-time order tracking and inventory management, helping logistics providers maintain efficiency in order management. Accurate data capture aids in forecasting and planning, thus playing a key role in maintaining an agile and responsive supply chain.
Challenges and the Future of OCR
In the evolving landscape of Optical Character Recognition technology, the challenges it faces and the anticipated future advancements are pivotal for understanding how OCR will continue to shape data capture solutions.
Limitations and Areas for Improvement
Though OCR technology has significantly advanced, accuracy remains a concern, particularly when interpreting documents with complex layouts, diverse fonts, or poor image quality. Human error in the data preprocessing stage can exacerbate these issues, leading to suboptimal outcomes. There's a continuing need for improvement in contextcomprehension, as OCR systems often struggle to understand the meaning behind the text they capture, making mistakes that a human wouldn't.
Advancements in Artificial Intelligence (AI) and Natural Language Processing (NLP) promise to address some of these shortcomings. By integrating Intelligent Character Recognition (ICR), systems can learn to interpret various handwriting styles and improve over time with machine learning algorithms. Nevertheless, the integration of such technologies with existing OCR tools through APIs is not always straightforward, presenting its own set of challenges for developers and users of data capture solutions.
Emerging Trends in OCR Technology
The future of OCR technology is rapidly intertwining with AI and machine learning. One can expect improvements in OCR systems through the incorporation of NLP, allowing machines to decipher the context and meaning of text with greater precision. This evolution is particularly significant for fields that rely heavily on nuanced data interpretation, such as legal and medical document analysis.
OCR solutions are also looking to offer more robust APIs that enable seamless integration with various business applications, expanding the versatility and applicability of OCR in different industries. This interconnectivity will likely streamline workflows and increase the efficiency of data-centric tasks. As OCR technology advances, it will become an even more essential part of data capture solutions, evolving beyond character recognition into a tool that provides comprehensive, actionable insights from the textual data it processes.